Annotation Tools for Machine Learning: Empowering Businesses
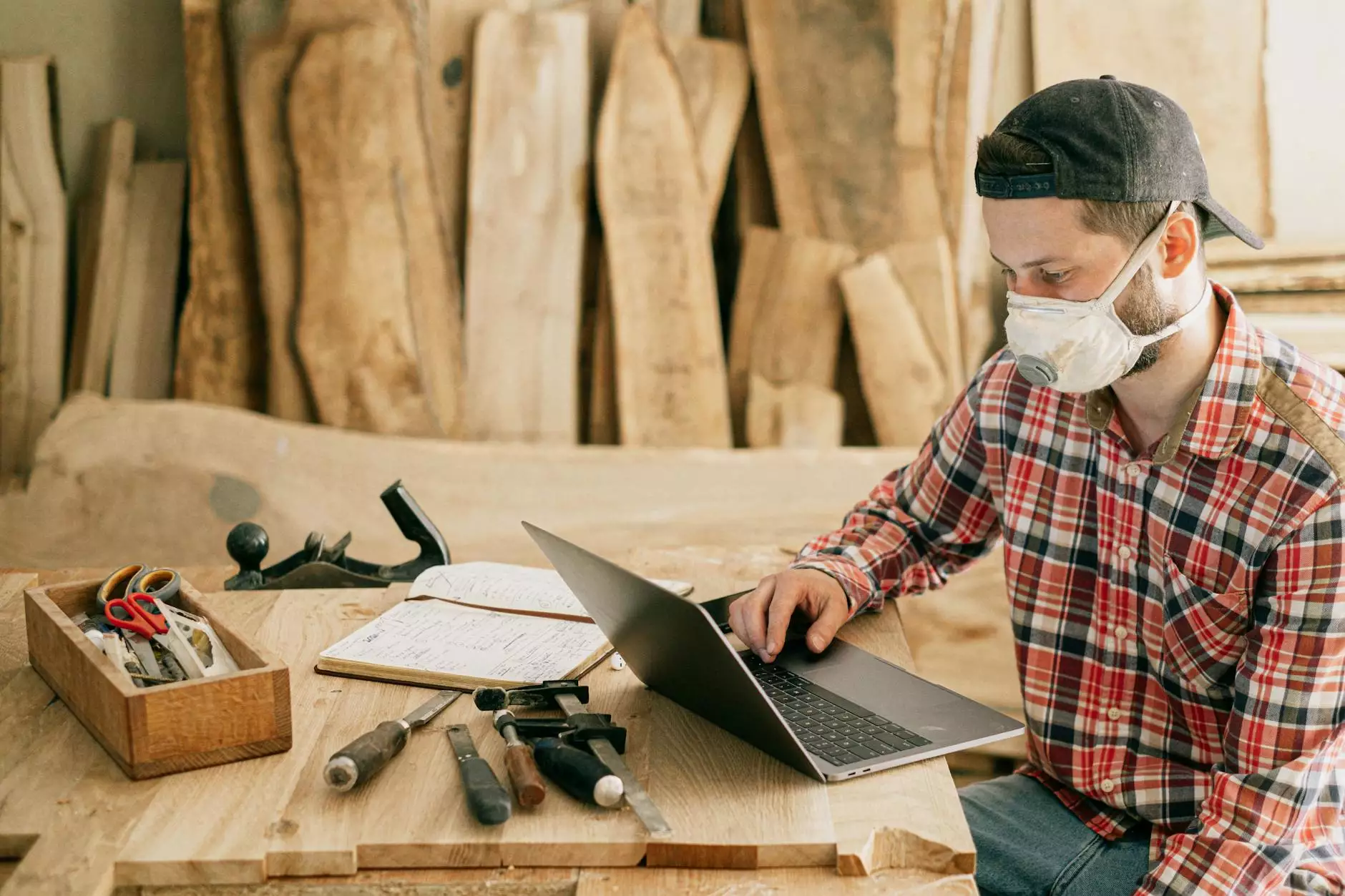
The digital landscape is continuously evolving, and businesses are progressively turning towards machine learning to gain a competitive advantage. One of the critical components of successful machine learning applications is the effective annotation of data. In this article, we will delve deep into annotation tools for machine learning, their significance, types, and impact on various business sectors, especially in the realm of Home Services and Keys & Locksmiths.
Understanding Machine Learning and Data Annotation
Machine learning (ML) is a subset of artificial intelligence that allows systems to learn and improve from experience without explicit programming. At the heart of machine learning models lies data, which must be accurately labeled or annotated to train algorithms effectively. This process is known as data annotation, involving tagging data with meaningful labels that enable machines to understand and interpret it.
The Crucial Role of Data Annotation
Data annotation is essential for the following reasons:
- Quality Model Training: Machine learning models require quality data to learn effectively. Annotated data provides the necessary context for algorithms.
- Improved Accuracy: Properly annotated datasets lead to higher accuracy in model predictions, which is crucial for businesses relying on data-driven decisions.
- Scalability: As businesses grow, the volume of data increases. Efficient annotation processes allow businesses to scale their ML efforts without compromising data quality.
Types of Annotation Tools for Machine Learning
Annotation tools for machine learning come in various forms, each tailored to specific data types and business needs. Below, we will explore the most prevalent types of annotation tools, how they function, and their applications in business.
1. Image Annotation Tools
Image annotation tools are used to label images for tasks such as object detection, image segmentation, and labeling facial expressions. These tools allow businesses in sectors like Home Services to train models that can analyze images related to service requests, customer identification, or property inspections.
- Bounding Boxes: This technique involves drawing boxes around objects within an image.
- Polygon Annotation: Useful for irregular shapes, polygon annotation provides more precise labeling of objects.
2. Text Annotation Tools
Text annotation tools help in marking up text data, useful for natural language processing (NLP) tasks. These tools can classify sentiments, entities, and keywords, invaluable in customer service or marketing analytics.
- Named Entity Recognition: Identifying and classifying key elements in text.
- Sentiment Analysis: Evaluating the tone of a text to better understand customer feelings.
3. Audio Annotation Tools
Audio annotation tools are used to label audio clips, essential for speech recognition models. For businesses in Home Services and Keys & Locksmiths, these tools can help in analyzing customer calls or service interactions.
- Transcription: Converting spoken words into written text.
- Speaker Diarization: Distinguishing between different speakers in a conversation.
Benefits of Using Annotation Tools
Utilizing annotation tools for machine learning presents various advantages for businesses, which can enhance operational efficiency and customer satisfaction. Here are several key benefits:
1. Increased Efficiency
Automated annotation tools can significantly reduce the time required to label large datasets. This efficiency allows businesses to concentrate on higher-value tasks rather than labor-intensive data labeling.
2. Enhanced Accuracy
Manual annotation is often prone to human error. Annotation tools, particularly those powered by AI, can help minimize mistakes and improve the overall quality and reliability of the datasets being used for model training.
3. Cost-Effectiveness
By streamlining the annotation process, businesses can reduce costs associated with data preparation. This is particularly critical for small and medium enterprises in the Home Services sector.
4. Support for Diverse Data Types
Many annotation tools support a variety of data formats—images, text, audio, and even video. This flexibility means businesses can handle multiple forms of data without needing different tools, promoting a cohesive approach to data management.
How to Choose the Right Annotation Tool for Your Business
Selecting the appropriate annotation tool for machine learning requires careful consideration of your specific business needs. Here are essential factors to consider:
1. Type of Data
Identify the type of data that will require annotation—images, text, audio, or a combination of these. Choose a tool that specializes in your primary data format.
2. User-Friendliness
A tool with an intuitive interface can save time and reduce frustration for your team. Look for options that require minimal training to use effectively.
3. Scalability
Consider whether the tool can scale with your business as data needs grow. The ideal tool should accommodate increasing amounts of data without loss of performance.
4. Budget
Budget constraints are a reality for many businesses. Evaluate the cost of the tool against its features and the potential return on investment it can provide through improved data handling.
Implementing Annotation Tools in Your Business
Successfully integrating annotation tools for machine learning into a business requires a strategic approach. Here are key steps to ensure a smooth implementation:
1. Assess Your Data Needs
Conduct a thorough analysis of your data requirements to identify which datasets need annotation and how they will be used in your ML models.
2. Train Your Team
Invest time and resources in training your staff on how to use the chosen annotation tools effectively. Proper training can significantly enhance tool adoption and productivity.
3. Monitor and Adjust
After implementation, continuously monitor the performance of your annotation processes. Be prepared to adjust strategies or tools as necessary to optimize outcomes.
4. Collect Feedback
Regularly gather feedback from users to identify any challenges they encounter. This feedback can inform improvements to your data annotation practices.
Conclusion
In an era where data drives business decision-making, annotation tools for machine learning play a vital role in enhancing the effectiveness of machine learning applications. For businesses in the Home Services and Keys & Locksmiths sectors, leveraging these tools can lead to improved operational efficiency and customer satisfaction. By understanding the types of tools available, the benefits they offer, and how to implement them, you can position your business for success in the competitive landscape of machine learning.
As companies increasingly transition into data-driven models, the right annotation tool can make all the difference. Whether you're looking to enhance your customer service offerings, streamline operations, or gain insights into consumer behavior, investing in top-quality annotation tools is essential. Embrace this transformative technology and unlock the potential of machine learning for your business today!