The Transformative Power of Agriculture Datasets for Machine Learning
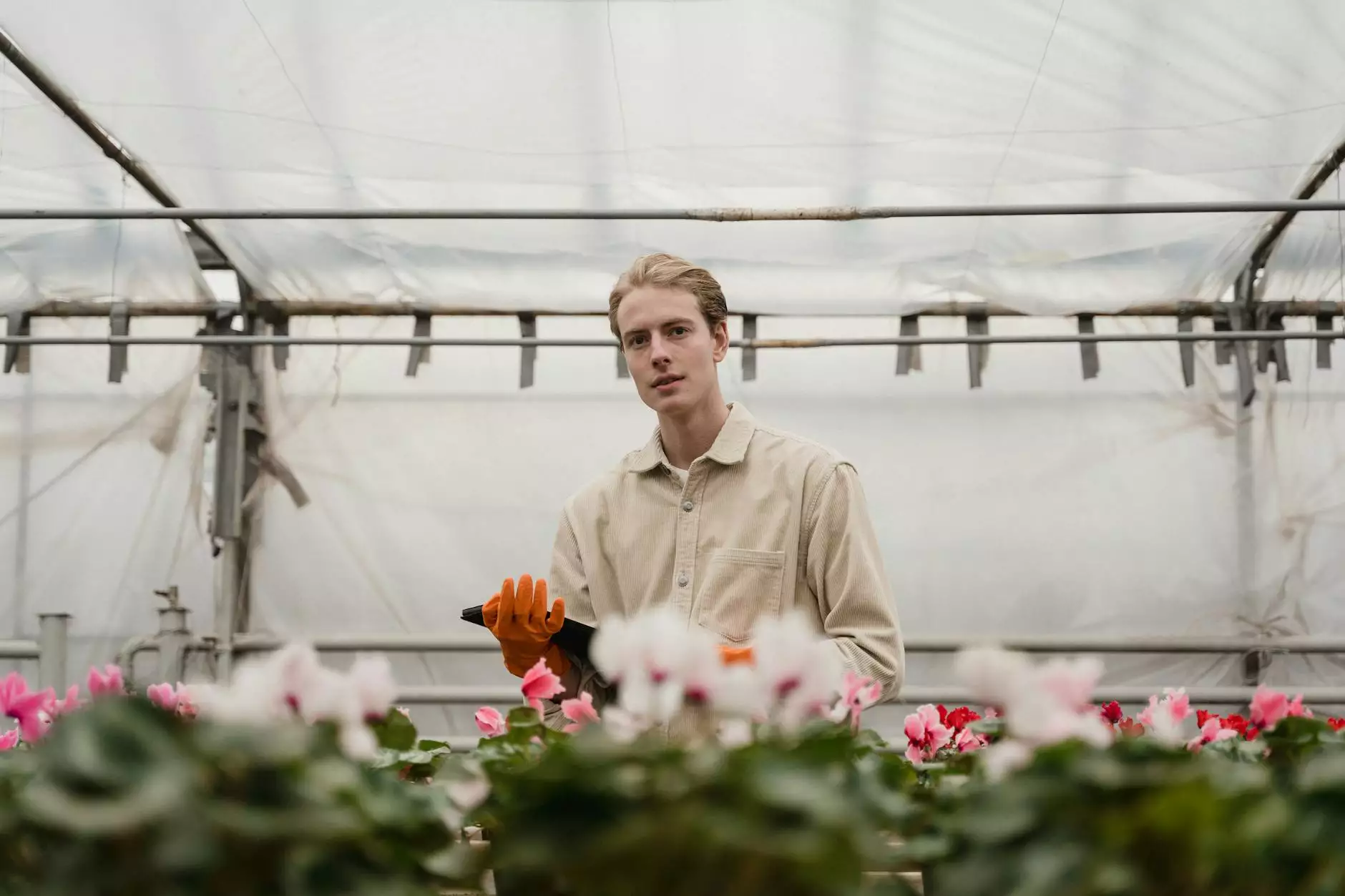
The intersection of technology and agriculture is rapidly evolving, catalyzing a revolution in the farming industry. One of the most promising advancements in this domain is the use of agriculture datasets for machine learning. This powerful combination is enabling producers to make informed decisions, optimize crop yields, and enhance sustainability practices. In this article, we will explore the significance of agriculture datasets in machine learning and how they are reshaping the agricultural landscape.
Understanding Agriculture Datasets
Agriculture datasets comprise a collection of data points related to farming practices, crop production, soil conditions, climate variables, and more. These datasets can be derived from various sources, including:
- Remote Sensing: Utilizing satellites to gather information on land use, moisture levels, and crop health.
- IoT Sensors: Deploying Internet of Things devices to monitor soil moisture, temperature, and nutrient levels.
- Historical Records: Analyzing past agricultural activities for trends and patterns.
- Field Surveys: Conducting manual assessments and data collection in agricultural fields.
The Role of Machine Learning in Agriculture
Machine learning (ML) refers to a subset of artificial intelligence (AI) that enables systems to learn and improve from experience without being explicitly programmed. In agriculture, ML algorithms analyze complex datasets to find patterns, make predictions, and recommend actions. The introduction of agriculture datasets for machine learning facilitates a myriad of applications:
1. Precision Agriculture
Precision agriculture leverages data-driven decisions to optimize farming efficiency and productivity. Through agriculture datasets, farmers can identify:
- Pest infestations and crop diseases.
- Water usage efficiency and irrigation needs.
- Soil nutrient deficiencies and fertilization schedules.
2. Crop Yield Prediction
With appropriately curated agriculture datasets for machine learning, farmers can predict crop yields with impressive accuracy. Predictive models can analyze variables such as weather conditions, historical yield data, and soil health to forecast potential outputs, enabling effective planning and resource allocation.
3. Supply Chain Optimization
Machine learning can also streamline the agricultural supply chain. By analyzing data related to market demand, transport logistics, and seasonal variations, stakeholders can enhance distribution strategies and minimize waste.
Key Benefits of Agriculture Datasets in ML
The utilization of agriculture datasets for machine learning provides several compelling benefits:
1. Enhanced Decision-Making
Data-driven insights lead to improved decision-making processes, mitigating risks associated with guesswork. Farmers can utilize the analyses to:
- Select the best crop varieties.
- Optimize planting schedules.
- Manage resources more effectively.
2. Increased Productivity
By harnessing the power of machine learning, farmers can achieve more significant yields per acre. The integration of relevant datasets allows for:
- Real-time monitoring of crop health.
- Early detection of pests and diseases.
- Timely interventions that improve overall productivity.
3. Sustainability and Environmental Impact
Utilizing agriculture datasets helps promote sustainable practices. By analyzing data related to soil health and resource usage, farmers can minimize their carbon footprint and preserve the ecosystem.
Challenges in Integrating Agriculture Datasets with Machine Learning
While the benefits are significant, there are several challenges that must be addressed when integrating agriculture datasets for machine learning:
1. Data Quality and Availability
Low-quality data can lead to inaccurate results. Reliable sources of data must be established to ensure that the models represent real-world conditions accurately. Additionally, data access can be limited, especially in developing regions.
2. Skill Gap
The integration of machine learning into agriculture requires expertise that might not be readily available. Training and education are critical to help farmers understand and utilize these technologies effectively.
3. Infrastructure Development
Agriculture, particularly in rural areas, may lack the necessary technological infrastructure, including internet connectivity and computing resources. Addressing these infrastructure gaps is essential for widespread adoption.
Case Studies: Successful Implementation of Agriculture Datasets in Machine Learning
1. John Deere: Smart Farming
John Deere, a leader in agricultural machinery, has incorporated machine learning into its equipment. By analyzing data from their machinery, farmers are provided with analytics that help optimize field operations, thus enhancing productivity and reducing costs.
2. Climate Corporation: Data-Driven Farming
The Climate Corporation employs advanced data analytics and machine learning to offer farmers predictive insights and tailored recommendations. This data-backed approach enables farmers to make informed decisions that lead to increased efficiency and profitability.
The Future of Agriculture Through Machine Learning
As the world population continues to grow, the demand for agriculture will inevitably rise. Leveraging agriculture datasets for machine learning paves the way for more efficient, productive, and sustainable farming practices. Here are some future trends to watch:
1. Advanced Predictive Analytics
Future developments in machine learning algorithms promise even more sophisticated predictive analytics, helping farmers anticipate changes in weather and market dynamics.
2. Improved IoT Integration
As IoT devices become more prevalent, the integration of real-time data collection into machine learning models will enhance decision-making processes further.
3. Collaborative Platforms
Collaboration among stakeholders—farmers, scientists, data analysts, and policymakers—will drive the development of comprehensive datasets and shared knowledge, fostering innovation in the agriculture sector.
Conclusion
The growing field of agriculture datasets for machine learning represents a pivotal opportunity in the agricultural industry. By leveraging advanced analytics and machine learning technologies, farmers can improve productivity, enhance sustainability, and make data-driven decisions that lead to successful farming practices. With ongoing advancements and the continued development of robust datasets, the future of agriculture looks promising, paving the way for a more efficient and sustainable approach to feeding the world.
To stay updated on the latest trends in agriculture, technology, and data analytics, visit us at keymakr.com.
agriculture dataset for machine learning