About Machine Learning: Transforming the Business Landscape
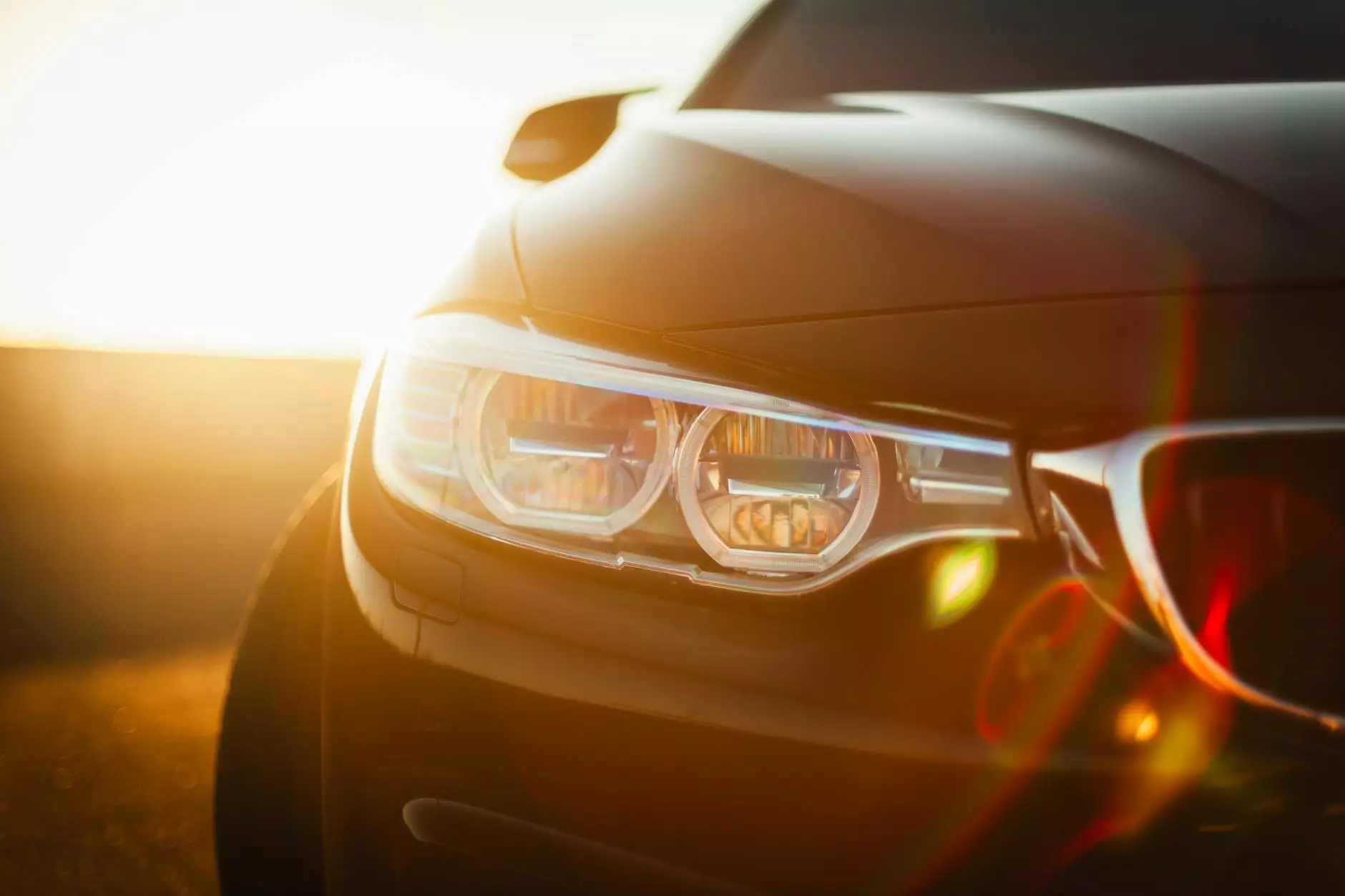
Machine learning is no longer just a buzzword; it has become a fundamental aspect of modern business operations. With its ability to analyze vast amounts of data and uncover hidden patterns, machine learning is revolutionizing how businesses understand their markets, optimize operations, and engage with customers. In this article, we will delve deeply into the core principles of machine learning, its applications across various industries, and the transformative effects it has on business strategy.
What is Machine Learning?
Machine learning is a subset of artificial intelligence that focuses on the development of algorithms that allow computers to learn from and make predictions based on data. It involves training a model on a dataset and then using that model to make decisions or predictions without being explicitly programmed for each task.
Key Concepts in Machine Learning
- Algorithms: The mathematical procedures that enable data processing and pattern recognition.
- Training Data: The dataset used to train the model, often containing labeled inputs and outputs.
- Model: The constructed representation that makes predictions based on trained algorithms.
- Testing Data: A separate dataset used to evaluate the model's performance after training.
Types of Machine Learning
Machine learning can be categorized into several types based on how learning occurs:
1. Supervised Learning
In supervised learning, models are trained on labeled data. This means that the input data is paired with the correct output, allowing the model to learn the relationship between the two. Regression and classification are common tasks in supervised learning. For instance, predicting sales figures based on historical data or categorizing emails as spam or not.
2. Unsupervised Learning
Unsupervised learning, in contrast, involves training on data without explicit labels. The model tries to identify patterns and group data points based on their similarities. A popular application of unsupervised learning is clustering, which groups customers based on purchasing behavior, allowing businesses to tailor their marketing strategies effectively.
3. Reinforcement Learning
Reinforcement learning is a type of machine learning where an agent learns to make decisions by interacting with an environment. It receives feedback in the form of rewards or penalties, allowing it to improve its performance over time. This approach is particularly useful in automated systems such as robotics and game playing.
The Importance of Machine Learning in Business
Machine learning offers significant advantages to businesses looking to maintain a competitive edge. Here are some critical benefits:
1. Enhanced Decision Making
Data-driven decision making is paramount for modern businesses. Machine learning enables organizations to analyze historical data and predict future trends. This allows for better resource allocation, risk management, and strategic planning.
2. Improved Customer Experience
By leveraging machine learning, businesses can personalize their offerings to meet customer preferences. Through techniques such as recommendation systems, companies can suggest products or services that are more likely to engage customers, leading to higher satisfaction rates.
3. Operational Efficiency
Automation powered by machine learning can streamline routine processes, minimizing human error and reducing costs. For instance, businesses can automate customer service inquiries using chatbots that learn from previous interactions.
4. Predictive Analysis
Predictive analytics, enabled by machine learning, can help businesses forecast market trends and consumer behavior. By understanding what customers are likely to do, companies can tailor their strategies accordingly, whether it’s inventory management or marketing campaigns.
Applications of Machine Learning Across Industries
Machine learning is versatile and applicable in various fields. Here are some prominent sectors that benefit from machine learning:
1. Healthcare
Machine learning is transforming healthcare by enabling the analysis of patient data to detect diseases earlier and improve treatment plans. For instance, medical imaging has improved drastically through machine learning algorithms that help radiologists identify abnormalities in scans with high accuracy.
2. Finance
The finance industry uses machine learning for fraud detection and risk assessment. By analyzing transaction patterns, machine learning models can identify suspicious activities in real time, thereby protecting businesses and consumers alike.
3. Retail
Retailers use machine learning to optimize inventory levels, manage supply chains, and enhance customer personalization. By analyzing purchasing trends, retailers can predict which products will be in demand and ensure they meet customer needs efficiently.
4. Manufacturing
In manufacturing, machine learning helps in predictive maintenance, allowing companies to forecast when machinery is likely to fail and schedule maintenance accordingly. This reduces downtime and maintenance costs.
5. Transport and Logistics
Machine learning algorithms optimize routing for delivery trucks and freight forwarding, leading to faster deliveries and reduced fuel costs. Companies like Uber and Lyft leverage machine learning to dynamically price rides based on demand patterns.
Challenges in Implementing Machine Learning
While the benefits of machine learning are significant, organizations face challenges in its implementation:
1. Data Quality and Availability
The effectiveness of machine learning models heavily relies on quality data. Organizations must invest in data collection and cleaning processes to ensure the accuracy of their datasets.
2. Technical Expertise
Implementing machine learning requires skilled professionals. Companies often struggle to find talent with the right expertise, which can slow down development and deployment.
3. Integration with Existing Systems
Integrating machine learning solutions into legacy systems can be complex. Businesses need to ensure that new technologies work seamlessly with existing infrastructure.
4. Ethical Concerns
Organizations must navigate ethical dilemmas, such as data privacy and algorithmic bias, to ensure responsible use of machine learning technologies.
Future Trends in Machine Learning
As we look ahead, several trends are poised to shape the future of machine learning in business:
1. Automated Machine Learning (AutoML)
AutoML aims to simplify the process of applying machine learning by automating steps traditionally requiring human expertise—making machine learning more accessible to a broader range of professionals.
2. Explainable AI
A growing focus on explainable AI seeks to make machine learning models more transparent. As businesses rely more on AI-driven decisions, understanding how those decisions are made becomes critical.
3. Edge Computing
With the rise of IoT devices, machine learning algorithms will increasingly be deployed at the edge of networks to allow faster data processing and reduce latency.
4. Enhanced Collaboration between Humans and Machines
The future will see more synergy between humans and machine learning systems, augmenting human decision-making capabilities rather than replacing them.
Conclusion
In conclusion, understanding machine learning and its implications for business is crucial for success in today's data-driven landscape. As organizations embrace this technology, they gain powerful tools to enhance operations, improve customer experiences, and drive innovation. By recognizing the challenges and leveraging the opportunities presented by machine learning, businesses can position themselves for long-term growth in an increasingly competitive market.
If you would like to learn more about how machine learning can transform your business strategies, visit us at machinelearningconsulting.net.
about machine learning