Labeling Tools for Machine Learning: A Comprehensive Guide
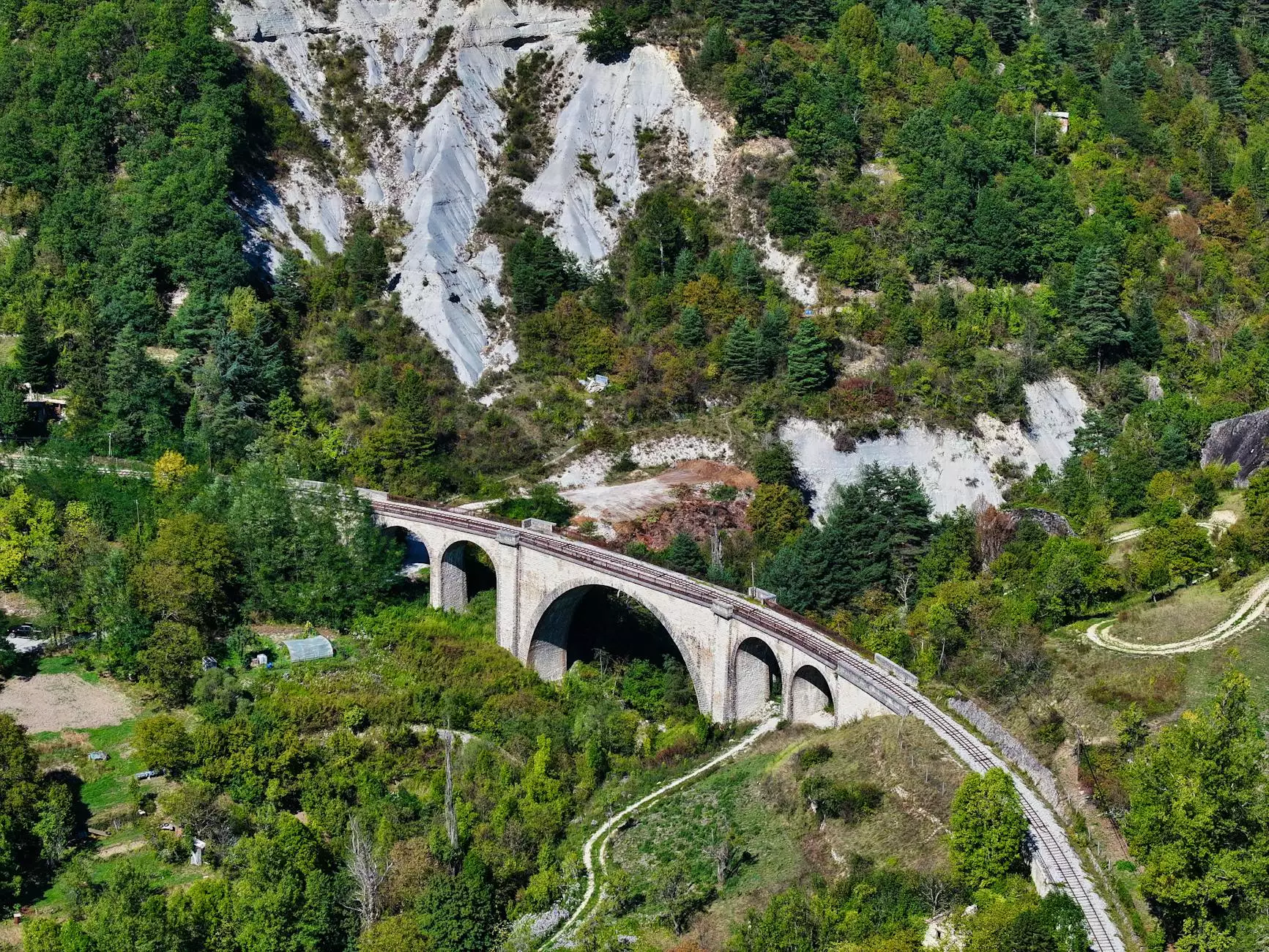
In the rapidly evolving world of machine learning, data is the cornerstone that drives the development and accuracy of algorithms. However, for machines to learn effectively, they need well-annotated datasets. This is where labeling tools for machine learning come into play. In this comprehensive article, we will delve into the significance of these tools, their features, benefits, and how they can transform your data annotation processes.
The Importance of Data Annotation in Machine Learning
In machine learning, data annotation is the process of labeling the data that will be used to train models. This can include anything from tagging images with descriptive keywords to categorizing text documents. The quality of the annotations directly influences the performance of the machine learning models. Poorly labeled data can lead to inaccurate models, rendering the results unreliable.
Data annotation is not just a one-time task; it is an ongoing process as new data is generated and collected. As such, having efficient labeling tools is crucial for ensuring that your dataset is accurate, comprehensive, and as free from bias as possible.
Key Features to Look for in Labeling Tools for Machine Learning
When choosing labeling tools for machine learning, it’s essential to consider several key features that can enhance your data annotation efforts. Here are some of the most important attributes to evaluate:
- User-Friendly Interface: The tool should be intuitive and easy to navigate, allowing annotators to focus on their tasks without any technical difficulties.
- Collaboration Capabilities: Look for tools that enable team collaboration, allowing multiple users to annotate data simultaneously.
- Customizable Annotation Templates: The ability to create custom templates can streamline the annotation process and ensure consistency across the dataset.
- Support for Various Data Types: The best tools support multiple data formats such as images, videos, audio, and text.
- Integration with Machine Learning Frameworks: Choose tools that can seamlessly integrate with popular ML frameworks like TensorFlow, PyTorch, and more.
- Quality Control Features: Features like review and audit trails are essential for maintaining high-quality annotations.
- Automation Support: Some tools offer automation features such as pre-labeling or machine-assisted labeling to speed up the process.
The Benefits of Using Labeling Tools for Machine Learning
Utilizing specialized labeling tools for machine learning offers numerous benefits:
1. Increased Efficiency
Automated features and a user-friendly interface can significantly reduce the time spent on data annotation, enabling faster model training cycles.
2. Enhanced Accuracy
High-quality tools can help minimize human error in data labeling, resulting in more precise and reliable datasets.
3. Scalability
As your project grows, having effective labeling tools allows you to scale your annotation efforts without sacrificing quality.
4. Cost-Effectiveness
By streamlining the annotation process, businesses can save on costs by reducing the time required for data preparation.
5. Improved Team Collaboration
With collaborative features, teams can work together efficiently, share feedback, and ensure that quality is maintained throughout the process.
Top Labeling Tools for Machine Learning
Several labeling tools stand out in the market for their unique features and capabilities. Below, we detail some of the top options available today:
1. KeyLabs AI Data Annotation Tool
The KeyLabs AI Data Annotation Tool is a powerful platform that encompasses a comprehensive suite of features designed for annotating various data types. Its user-friendly interface allows for both manual and automated annotations, making it suitable for projects of all sizes. KeyLabs AI excels in integrating with existing workflows, allowing seamless adoption and immediate improvements in efficiency.
2. Labelbox
Labelbox is renowned for its versatility and collaboration capabilities. It supports a wide array of data formats and provides advanced annotation tools, such as segmentation and polygon annotation. Its built-in quality control features help maintain high standards across the dataset.
3. Supervisely
Supervisely is ideal for users working with computer vision projects. It offers an extensive set of tools for image and video annotation, along with powerful model training capabilities. Teams can rapidly annotate and review data, which accelerates project timelines.
4. Amazon SageMaker Ground Truth
Amazon SageMaker Ground Truth provides a scalable data labeling service integrated into the Amazon Web Services (AWS) ecosystem. It allows users to build highly accurate training datasets with both human and machine assistance, making it a robust option for enterprises with large datasets.
5. Snorkel
Snorkel introduces a unique approach to data labeling by allowing users to create labeling functions that programmatically generate labels for data points. This flexibility is particularly beneficial when combined with human-in-the-loop approaches for ensuring dataset quality.
Best Practices for Using Labeling Tools
To maximize the effectiveness of your labeling tools, consider the following best practices:
- Define Clear Annotation Guidelines: Establish clear standards and guidelines for your annotation tasks to ensure consistency and quality.
- Train Your Team: Invest time in training your team on the annotation tool and processes to minimize errors and improve efficiency.
- Regularly Review and Audit Annotations: Implement a regular review process to catch errors early and maintain high-quality datasets.
- Utilize Pre-labeling Features: Take advantage of automation features offered by modern tools to streamline the initial labeling process.
- Gather Feedback: Encourage your annotation team to provide feedback on the tools and processes for continuous improvement.
Future Trends in Data Annotation for Machine Learning
As the machine learning landscape continues to grow, the role of labeling tools will evolve with it. Here are a few trends to watch for in the coming years:
1. Greater Automation
As machine learning technologies advance, we can expect labeling tools for machine learning to incorporate more automation features. These features will help reduce manual effort while still ensuring quality annotations.
2. Integration with Artificial Intelligence
The integration of AI in labeling tools will allow for smarter pre-labeling and predictive tagging, resulting in faster annotation cycles and improved accuracy.
3. Enhanced Collaboration Tools
As remote work becomes more common, tools that facilitate real-time collaboration among annotators will become increasingly important, ensuring teams can work seamlessly regardless of their location.
4. Focus on Data Privacy and Ethics
With rising concerns regarding data privacy and ethics, future labeling tools will likely incorporate features that help ensure compliance with regulations and ethical standards during the data annotation process.
Conclusion
In conclusion, the relevance of labeling tools for machine learning cannot be overstated. With their ability to enhance efficiency, accuracy, and collaboration, organizations that leverage these tools are better positioned to succeed in the data-driven world. As data continues to play a pivotal role in the performance of machine learning models, investing in high-quality labeling tools will be essential for any business aiming for success in artificial intelligence and machine learning initiatives.
Choosing the right data annotation tool and leveraging best practices will ensure your machine learning projects achieve the highest levels of performance and reliability. We encourage businesses to explore the various options available and identify the best fit for their unique needs.